Insights
The Role of Technology in Modern Clinical Data Management
30 Oct, 20238 minutesThe field of Clinical Data Management is undergoing significant modernisation and digitalisa...
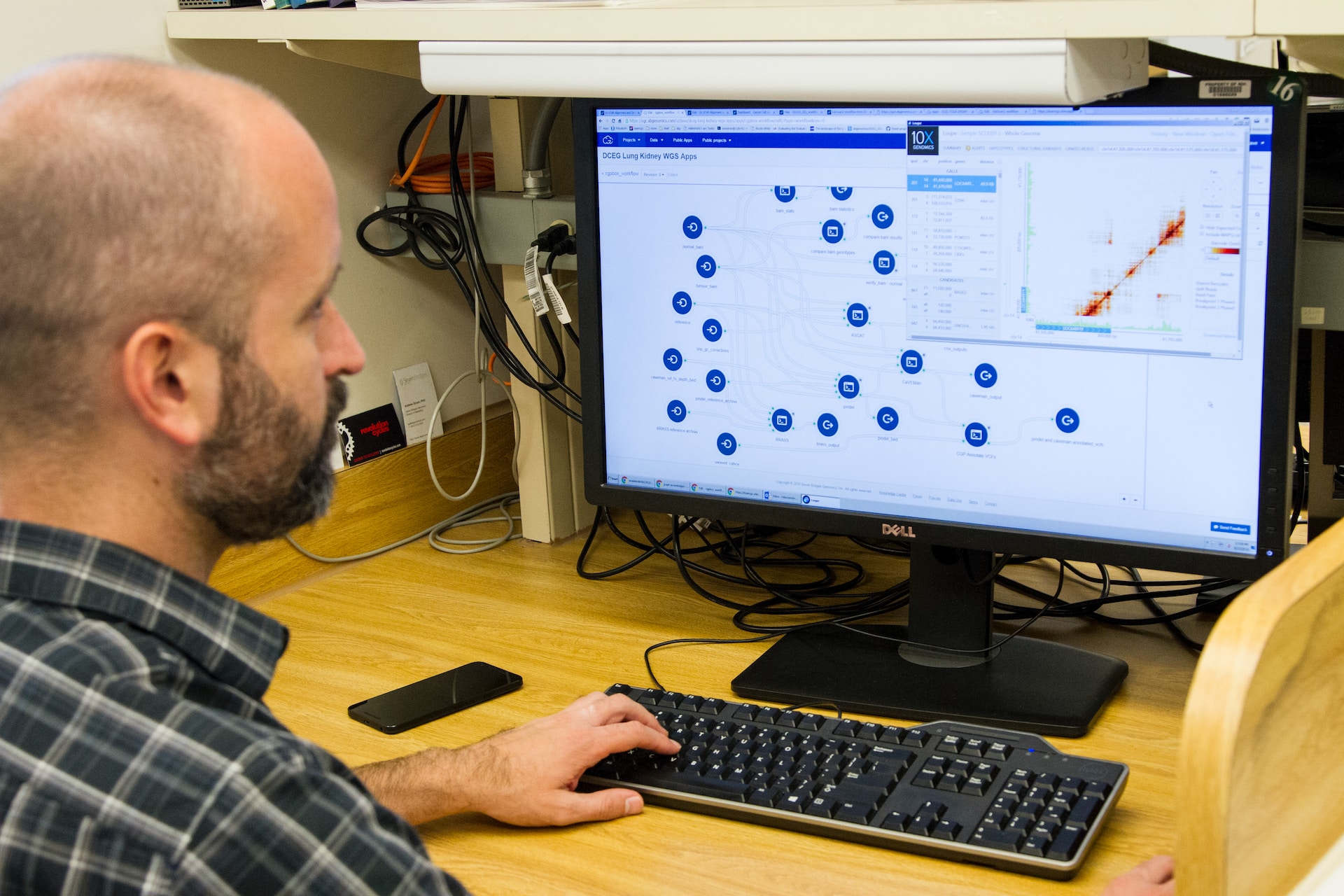
The field of Clinical Data Management is undergoing significant modernisation and digitalisation. No strangers to advancement, Statistical Programming and CDM experts are leveraging refinements in artificial intelligence and machine learning (AIML) technologies to enhance the strategic role they play across the entire trial process.
This guide will explore the latest technological trends revolutionising work across the entire clinical data science life cycle. From how Telemedicine empowers decentralised clinical trials to the growing utilisation of real-time and real-world evidence (RWE), Statistical Programming and CDM roles increasingly ensure the accuracy and quality of biometric information recorded and analysed throughout the trial process.
We’ll also discuss how these modernisations are driving a transformation in Clinical Data Science recruitment, with roles across Biometrics evolving in response to technological innovation.
Technological Trends Revolutionising Clinical Data Management
Over the last ten years, digital technology and computing power advancements have allowed Clinical Research Organisations (CROs) of all sizes, from startups to global market leaders, to rapidly evolve.
From the utilisation of artificial intelligence and machine learning to enhance the productivity of Clinical Data Management teams to the development of wearable medical devices that enable the collection of real-time data and real-world evidence (RWE), opportunities abound for CDM specialists to modernise the practice of data collection, analysis, and reporting.
So, what are the technologies and techniques that are helping to redefine Data Management and Statistical Programming roles?
1. Telemedicine: the Transformation of Decentralised Clinical Trials
According to figures reported in 2022, over one thousand decentralised clinical trials (DCTs) took place in 2021. This was the greatest increase in the virtual — or direct-to-participant — trials market to date.
Char Kumarage, our Practice Lead in Clinical Research, commented: "The Covid-19 pandemic was the catalyst that enabled DCTs to gain traction and compelled the pharmaceutical industry to innovate. With CRAs unable to visit patients in person, technology became pivotal, enabling patients to play a more active role in data collection. DCTs offer improved access for rural patients, increased patient diversity, and better retention, thanks to data collection in patients' homes."
Driving this transformation is the broader understanding within the clinical environment that trial participants were travelling an average distance of 67 miles, one way, to visit trial sites in 2022. In research conducted by Greenphire, a leading provider of financial solutions for Clinical Research Organisations, analysts noted that distance was likely to impede the recruitment and retention of clinical trial participants, particularly in cases of rare disease research and drug development. However, the situation is changing in 2023.
Char continued: "It is easy to see why DCTs gained popularity during this time, due to lack of alternatives. As the market has now stabilised and the industry is gradually returning to the traditional on-site monitoring model, revealing challenges in DCTs:
1. Data integrity: participants are trusted to gather data. They are not clinically trained so there is a probability of data being compromised and inaccurate.
2. Layoffs in DCT Facilitating Companies: Companies like Science 37 and Curebase have recently laid off staff, indicating a decreasing demand for DCTs.
3. Technology-related obstacles. Internet connectivity issues in rural areas and tech literacy problems among patients can compromise data collection."
2. Real-World Evidence: Enhancing Accuracy and Guiding Public Health Interventions
The COVID-19 pandemic highlighted the potential of mobile technologies to empower trial participants to share their health data and contribute towards generating real-world evidence. As a result, Clinical Data Management experts are able to capture, analyse, and report on long-term trends and patterns in drug or device efficacy and safety in settings outside of the trial site, complementing the data captured during other parts of the clinical data science life cycle.
Patient-reported outcomes (PROs) and biometric data collected between visits to trial sites—in the case of hybrid clinical trials—mean that statistical programming and clinical data professionals are able to ensure that trial participants’ perspectives are reported on. This offers insights into the impact of treatments on their everyday lives whilst reducing recall bias, and enhances the accuracy of data and reports.
These PROs are also becoming increasingly recognised by regulatory authorities as important tools for understanding how to label pharmaceutical products, whilst capturing subjective experiences—such as variable pain levels, overarching quality of life, and trial satisfaction—can influence public health policies.
3. Automation: Improving the Productivity of Clinical Data Specialists
Machine learning and artificial intelligence empower Clinical Data Management experts and Statistical Programmers alike, helping reduce the effort and time required to manage, analyse, and secure trial participants’ biometric data.
The growing popularity of these emerging technologies is allowing researchers and those involved in the Clinical Data Science life cycle to not only focus on their more complex, high-level responsibilities, but also help to speed up decision-making within the broader clinical trial environment and eliminate the potential for human error associated with manual data entry. AIML algorithms can automate the time-intensive practice of data cleaning and standardisation, improving consistency and aiding analysts to more quickly parse information and produce reports.
Similarly, Clinical Data Management automation tools are also increasingly able to monitor and identify insights in historical data, allowing Data Managers and Statistical Programmers to address problems with data quality before they impact the smooth running of the trial or regulatory approval.
Advancements in Natural Language Processing (NLP), powered by artificial intelligence and machine learning, are also able to extract, clean, and analyse the valuable unstructured data collected as part of the trial process, such as medical records and the aforementioned PROs, improving data accessibility and enhancing the overall research and reporting process.
This NLP software can even aid enrollment by identifying eligible trial participants by rapidly screening electronic health records. When research shows that 20% of US-based Oncology trials fail to meet their participant acquisition and retention targets, this is a significant step forward.
4. Interoperability: Enabling the Seamless Exchange of Clinical Data
By enabling the rapid and seamless communication of data between different Clinical Data Management systems, the increasing interoperability of Clinical Decision Support Systems (CDSS), Health Information Exchanges (HIEs), Electronic Health Records (EHRs), and medical devices allows statistical programming and data professionals to access participant data and collaborate effectively.
Interoperability encourages the standardisation of data and the agreement between laboratories, clinical trial sites, pharmacies, hospitals, and medical practices to share participant information and ensure safeguards are in place to keep that information safe.
This is crucial within the Clinical Data Management sphere since it improves efficiency, enhances participant safety in the case of adverse effects, and supports wider efforts towards digitalisation and telehealth across the life science and public health sectors.
Alongside this, interoperability often leads to reductions in the cost of administering and analysing the biometric data generated during the clinical trial process, enhancing trial coordination and minimising errors in data by reducing the need for manual data entry. This is because data is presented consistently across all software platforms and paperwork utilised throughout the trial, enabling professionals to quickly access and leverage participant information in decision-making.
5. Risk-Based Quality Management (RBQM): Data-Driven Enhancement of Trial Safety
Risk-based quality management (RBQM) technology is playing an increasingly important role in the development of Clinical Data Management jobs.
Whilst RBQM is a wider methodology in clinical trials, several software packages and techniques have risen out of the practice, such as Centralised Statistical Monitoring (CSM), enhancing quality oversight by analysing data for discrepancies that might remain undetected by otherwise traditional Data Management techniques.
RBQM ensures that risk is mitigated when dealing with all forms of biometric data by allowing Statistical Programming and Data Science professionals to ensure the accuracy and completeness of data sets.
In terms of software interoperability, RBQM requires all front-end user interfaces to be intuitive and ‘system-agnostic’, ensuring that trial staff find it easy to use and can integrate it with their existing systems. This allows for the fast reporting of trial data, speeding up progress and reducing costs by providing Trial Coordinators, Managers, and Directors with better insights.
As technology and computing power improve, new software and Clinical Data Management tools suites will allow statistical programming and data professionals to collect, store, analyse, and report biometric data efficiently and quickly. The ongoing refinement of AIML means that analysts will be empowered to work with ever-larger data sets, ensuring that trials are able to target and represent demographics with more accuracy.
The overarching impact of these new technologies will be to improve drug development processes, enabling pharmaceutical organisations to release drugs and devices that target rare diseases with more efficacy, and CDM experts play a significant role in ensuring these therapies achieve regulatory approval and make it to market.
How These Trends Are Shaping Clinical Data Science Recruitment
As a result of the technologies we explored in our last section, we’ve seen the evolution of Clinical Data Management and Statistical Programming roles. So, how are they influencing Clinical Data Science recruitment at large?
Technologies such as AIML and increasing interoperability between the different forms of software and Clinical Data Management systems mean that CDMs are increasingly expected to come to the role with the capability for interdisciplinary work. As these technologies evolve, we anticipate that additional responsibilities will be added to the everyday duties that CDM and Statistical Programming professionals carry out. CDMs are playing a larger part in guiding the activities of other trial teams, with many going on to lead and coordinate trials themselves.
At the same time, Statistical Programming roles are being reshaped into a more strategic position. As a PhUSE paper authored by Sascha Ahrweiler reports, these roles might be better referred to as Clinical Data Scientists over time, requiring additional skills in Biostatistics, CDM, and Operations.
Given Statistical Programmers' already critical role in overall trial success, these additional responsibilities will allow ambitious professionals to play a major part in healthcare and pharmaceutical advances.
With the growing adoption of risk-management and RBQM methodologies, Clinical Data Managers and Statistical Programmers will increasingly be able to identify and notify Trial Coordinators and Clinical Safety Managers of issues at any point in the trial process, minimising the possibility of losing participants.
Economists expect the global market for Clinical Data Management to grow by over 10% by 2028, driven by a growing need for data standardisation, the wider adoption of interoperable electronic data capture systems, the refinement of medical devices and telehealth leading to advances in real-time evidence acquisition, and a burgeoning market for software that supports the entire Clinical Data Science life cycle.
If you’re interested in learning more about how the CDM field is evolving, see our recent guide on How to Begin a Career in Clinical Data Management for a closer look at the value that these experts bring to their posts.
Key Findings
The fields of Clinical Data Management and Statistical Programming are undergoing a remarkable transformation due to the rapid integration of cutting-edge technologies. Across the clinical data science life cycle, the surge of innovation—including decentralised clinical trials, AI-driven tools that enhance Big Data analysis, and the growing use of real-time data and real-world evidence—are reshaping traditional practices.
These advancements are not only enhancing the accuracy, efficiency, and safety of data collection and analysis, but are also significantly influencing data science recruitment strategies. The evolution of Clinical Data Management roles is particularly evident, with many employers within the life science environment expecting interdisciplinary skill sets from their new hires.
Furthermore, with increasing interoperability facilitating the seamless exchange of data throughout the clinical trial environment and RBQM adding an additional layer of accuracy and vigilance to data management positions, these trends are encouraging Programmers and Data Managers to play a pivotal role in advancing healthcare and pharmaceutical innovation.
With a surge of demand for data standardisation, real-time evidence acquisition, and sophisticated software across the clinical data science life cycle, the further of the field promises further progress and impactful research.
Sourcing Clinical Data Management Specialists
Warman O’Brien maintains a presence within the UK, US, and Asia Pacific Regions, aiding professionals in securing cutting-edge Statistical Programming, Clinical Trial Data management, and Biostatistics jobs with market-leading life science organisations.
Our consultants have a solid track record of providing expert talent solutions for a range of vacancies, and can help to address your executive search and high-volume staffing needs. We regularly present at conferences such as PHUSE, PharmaSUG, and the American Society of Gene & Cell Therapy’s annual meetings, which have enabled us to build an extensive network of global companies and expert candidates for the latest clinical research roles. Get in touch with us to discuss your hiring needs or career ambitions today.